Recent Posts
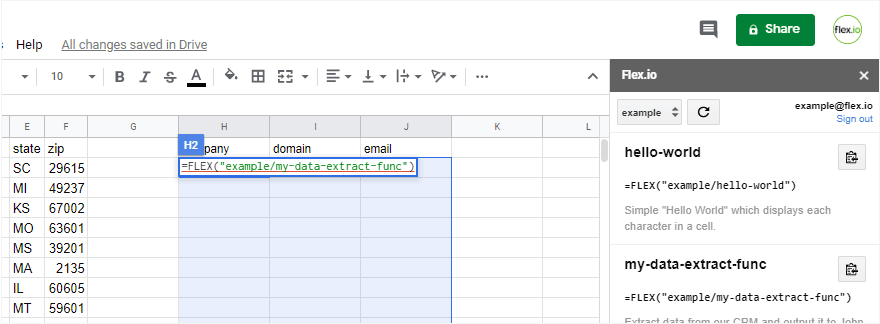
Integrating Web Services with Microsoft Excel and Google Sheets
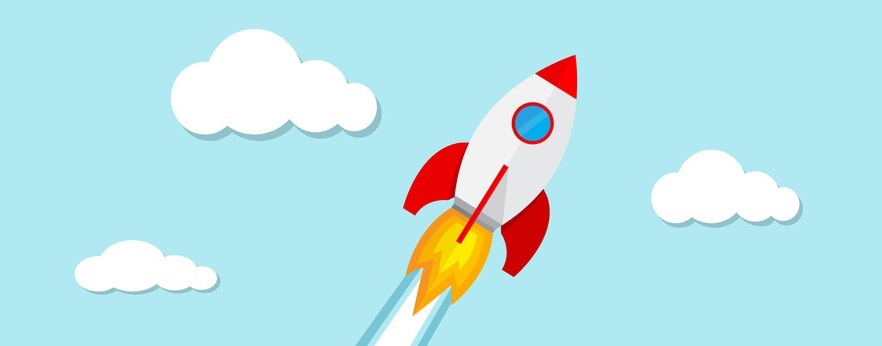
The "Aha" Moment: How to Onboard an API Service and Get Active Users
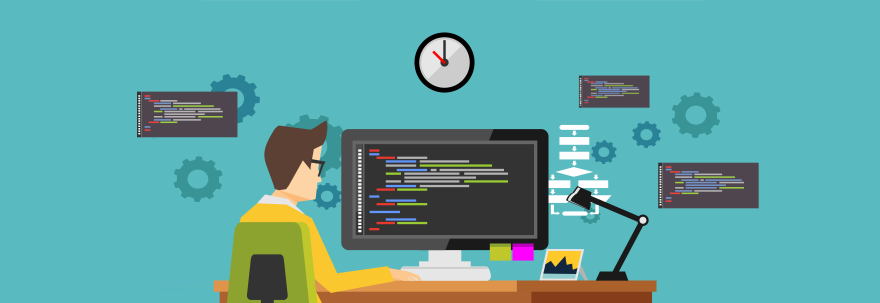
Introducing Serverless Data Feeds

Share Data Without Sharing Credentials: Introducing Pipe-level Permissions

Lessons from the Data Ecosystem: Part 2

What We've Learned from Exploring the Data Ecosystem: Part 1

Improving Data Access: Getting Past Step One

Data Prep to Data Pipes: 5 Facets of a Data Project

Let a Thousand Data Silos Bloom

5 Steps to Faster Data Projects

Decentralized Data Projects are the Norm
In an excellent post, CIO Isaac Sacolick asks, what technologies work best for decentralized data scientists?
It’s a great question. As he aptly describes it, working with data in a decentralized environment presents a challenging scenario:
But what happens when these resources are scattered across multiple departments. One department may have an expert data scientist, another may have a small group doing internal reporting, and a third group might have outsourced its analytic function. If data scientists in the organization are decentralized with different goals, skills, and operating models, can IT still provide a common set of Big Data and analytic tools and services to the organization and support these different functions?
I think this question is relevant even when IT is supporting a single group of data scientists in one location.
Most data projects are decentralized in one way or another. They involve a range of efforts between different groups, whether this involves setting up a data transfer or coordinating data-related work on different teams. It comes with the territory.
For instance, typically one of the first steps in a new project is chasing down the data. Data scientists don’t usually generate the source data they use; the data comes from some other group. So right at the start, there are multiple parties involved and all of the wonderful rituals that go with this – submitting the business case, requesting data dictionaries, trading email about the status of the data transfer, etc.

Since decentralization is a common feature of data projects, what’s a fitting approach for selecting the best tools for this work?
If only it was as simple as just upgrading existing BI infrastructure. However, a lot of data science is done outside the context of traditional BI, and the kinds of tools users might need could be very different than the legacy BI tools in IT’s current technology stack. And on top of that, the BI landscape is undergoing a radical transformation, which is producing a flood of new technologies – future BI infrastructure may be very different than it is today.
A better approach is to consider how to make data projects more efficient as a whole:
Optimize for productivity
The first thing to evaluate is the level of productivity gains you can get from better tools for your data science team. After all, the main point of analytic and data preparation tools is to help them produce meaningful results quickly – and this can have a significant payoff.
Streamline collaboration
Another key consideration is improving the interaction and communication among everyone involved, particularly between IT and data science teams. Simply eliminating the bottlenecks and delays in communication between groups can significantly improve the speed and efficiency of data projects.
Embrace an agile approach
Applying agile methodology to data projects makes life better for everyone – not only for data science teams but for IT as well:
Agile makes IT more agile. … The end goal is a broader information delivery “ecosystem” where high-quality and critical data is easily accessible to business users
With an agile approach, both IT and data science teams can move faster and iterate more quickly through project cycles. As a result, it becomes easier to respond more rapidly to business needs and to incorporate feedback from the business.
Recent Posts
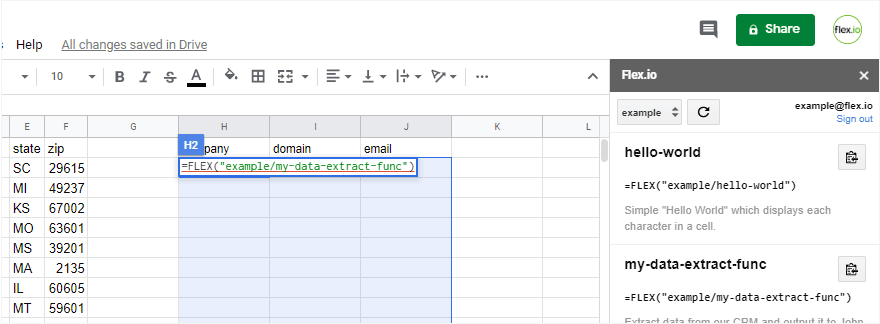
Integrating Web Services with Microsoft Excel and Google Sheets
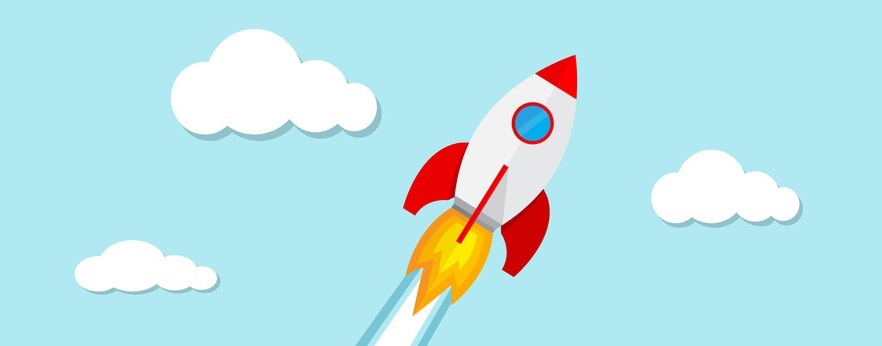
The "Aha" Moment: How to Onboard an API Service and Get Active Users
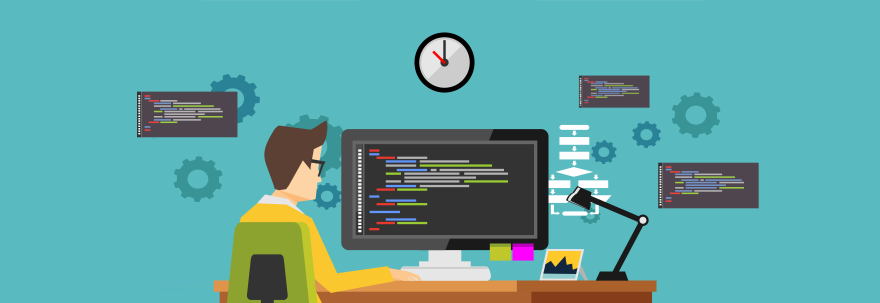
Introducing Serverless Data Feeds

Share Data Without Sharing Credentials: Introducing Pipe-level Permissions

Lessons from the Data Ecosystem: Part 2
