Recent Posts
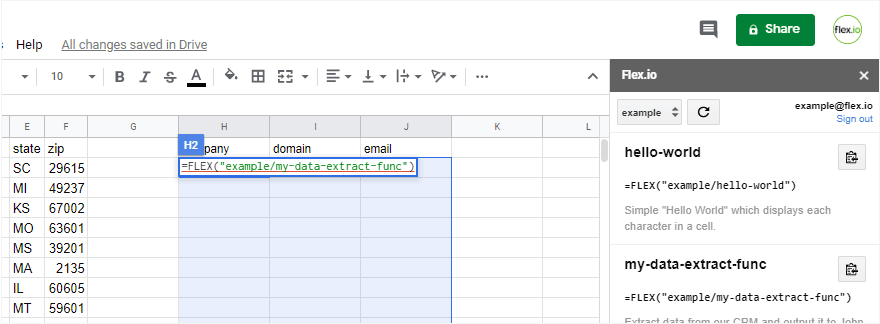
Integrating Web Services with Microsoft Excel and Google Sheets
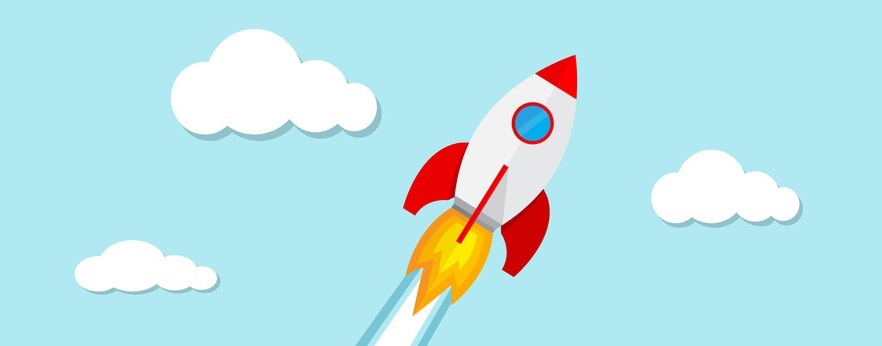
The "Aha" Moment: How to Onboard an API Service and Get Active Users
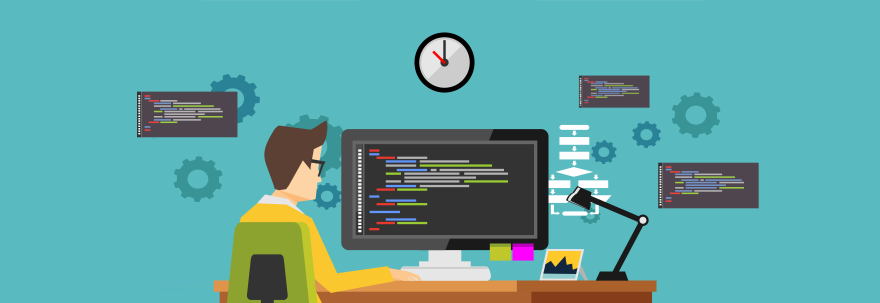
Introducing Serverless Data Feeds

Share Data Without Sharing Credentials: Introducing Pipe-level Permissions

Lessons from the Data Ecosystem: Part 2

What We've Learned from Exploring the Data Ecosystem: Part 1

Improving Data Access: Getting Past Step One

Data Prep to Data Pipes: 5 Facets of a Data Project

Let a Thousand Data Silos Bloom

5 Steps to Faster Data Projects

5 Steps to Faster Data Projects
Data projects come in many shapes and sizes. From big data predictive analytics to small data spreadsheet projects, from building new open data applications to reconciling a couple of ERP tables in the accounting department.
There’s one feature all these projects have in common: they all take far too long.
Efficiency matters – and for maximizing the value of data, it matters a lot. The math on this is simple. The more rapidly an organization can perform data projects, the greater its capacity to leverage data resources, generate real value and gain competitive advantage.
According to Gartner, more than half of analytic projects run over budget, break their schedule, or fail to meet expectations. This seems shocking at first, but once you start looking at the process involved, you can see why things go awry. As the ranks of CDOs grow and they work to improve the success rate of data projects, one key initiative should be to evaluate and improve the overall efficiency of the analytic workflow.

Here are five ways to improve the speed and efficiency of data projects:
1. Clarify Your Objectives
The key here is to know what you want to know.
Bringing clarity to your objectives provides a number of benefits. For instance, it makes it possible to identify the relevant data for the project and to define the final work product clearly. It makes it possible to select the best tools to utilize for the work and set a realistic schedule. It can even help determine the best way to communicate and present the results.
These days, everyone wants to jump on the data-driven bandwagon, but if there aren’t specific goals or metrics in mind from the start, it will almost certainly lead down a path of broken dreams and missed expectations.
2. Know Your Sources
It helps to know whether a data project is even feasible to begin with. Before you start, check to see if the source data is available and adequate to answer the questions involved. Maybe some essential data is missing. Maybe the data is too dirty or can’t be integrated in the way necessary for success. It’s best to find out about this up front, before investing a lot of time and resources in the project.
3. Apply the 80/20 Rule to Insights
Be opportunistic about what you want to know. Beware of questions that are of marginal value, but involve a significant investment of time and resources to answer. Focus on the analytic projects that can have the biggest impact with the least amount of effort involved.
Perhaps a senior manager puts in a request to “figure out what’s going on with X and Y”, but there are endless rabbit trails, data sets and analytic gymnastics required to identify every last immaterial line item. Once you’ve performed steps #1 and #2 above, you’ll be in a good position to pick your battles.
4. Identify and Remove Bottlenecks
Your analytic workflow is only as fast as the slowest step. Consider the time involved in initiating a new data project (e.g. developing business cases, allocating resources), gathering and preparing data resources for analysis and identifying accessible tools and team members.
Data requests alone can eat up a tremendous amount of time. For example, at the O’Reilly Strata conference earlier this year, the analytics director for a large company reported that just writing up the requirements for new data requests took up to 25% of her team’s time – a bottleneck they ultimately eliminated by adopting self-service BI tools.
5. Streamline Communication
Data projects are a team sport. As with any group effort, making it easier to communicate and collaborate helps everyone stay informed, coordinate activities and resolve issues.
Unfortunately, all too often, communication takes the form of spreadsheets or CSV files sent by email. This is great for generating huge email chains, but not so great for keeping track of all the moving parts in a project. And, a lack of source control makes it difficult to handle changes with the data and can lead to “many versions of the truth.”
Thankfully, project management software can be helpful here and more and more vendors are adding collaboration tools to their products.
. . .
Given the complexities of using data and the general inertia of organizations, data projects may never reach an ideal state of optimal efficiency. But by setting clear goals and doing some investigative work up front, you can certainly streamline the steps involved and get your data projects done faster.

Recent Posts
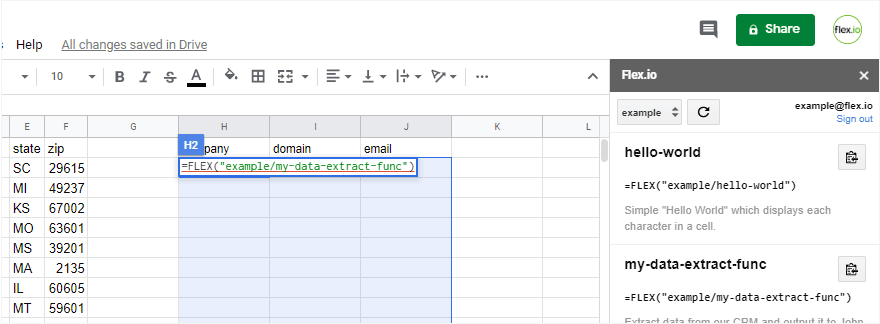
Integrating Web Services with Microsoft Excel and Google Sheets
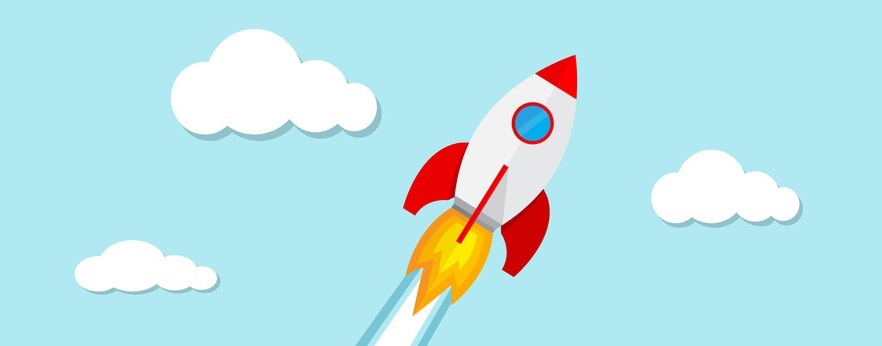
The "Aha" Moment: How to Onboard an API Service and Get Active Users
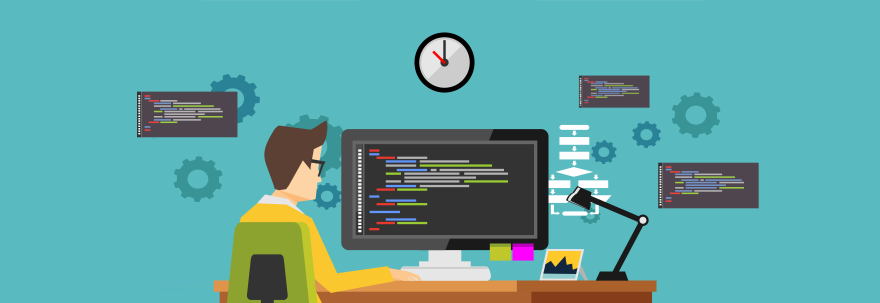
Introducing Serverless Data Feeds

Share Data Without Sharing Credentials: Introducing Pipe-level Permissions

Lessons from the Data Ecosystem: Part 2
